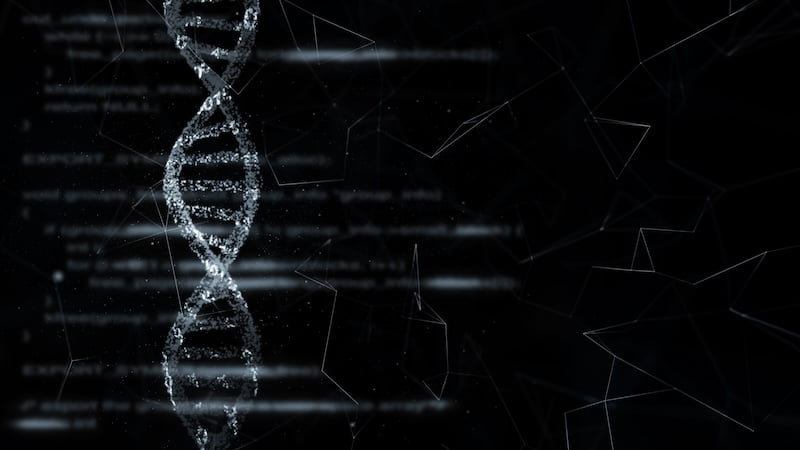
Large language models such as ChatGPT are currently the main tools for generating AI texts. However, an emerging problem in this area is the phenomenon of “text incest” – a term that describes the cyclical use of AI-generated text as training material for these models. In the following I want to deal with this problem.
Language models are trained on large datasets of human-written texts, drawn from a variety of sources such as books, articles, and websites. Through this training, they are able to produce texts that are very similar to human texts in syntax, context and creativity.
Text incest in AI language models: Background to the problem
However, as these models become more widespread, the internet is becoming more and more saturated with AI-generated content. If this content is now reused as training data, a kind of “feedback loop” is created that is referred to as “text incest”.
This term metaphorically refers to biological incest, where genetic information is recycled in a closed cycle, resulting in lower diversity and greater vulnerability.
Input equals output: The self-referential cycle in AI texts
The core of text incest lies in the self-referential cycle in which a language model is trained with its own output. This cycle can lead to various problems:
- Creating Echo Chambers: When a language model is constantly trained on the content it generates, it can become an echo chamber in which its existing biases and patterns are reinforced. This could limit the diversity of language and “thought” in the results.
- Weakening of creativity and novelty: One of the outstanding features of human language is its evolutionary character, characterized by creativity and novelty. Language models that are “trapped” in textual incest run the risk of producing rutted and predictable results that lack the very dynamic development observed in human language.
- Amplification of errors: If a language model accidentally produces factually incorrect or biased content and that content is used for further training, these inaccuracies can become compounded over time, leading to a decrease in the reliability of the model.
AI text incest: implications of the problem
The consequences of text incest first become apparent when it comes to the question of the reliability of information. The increasing prevalence of AI-generated content that may be inaccurate poses the risk of spreading misinformation.
This risk is particularly worrying because it can have a direct impact on areas such as education, journalism and public discourse. False or misleading information disseminated by such AI systems could have far-reaching consequences for information quality and trust in digital media.
Another issue is the stagnation of language development. Language in particular, which is characterized by dynamics and is strongly influenced by cultural, social and historical factors, could be affected by “language models in text incest”.
If a language model continually draws on its own previous results, it risks drifting away from the natural evolution and diversity of human language. This could not only lead to an impoverishment of linguistic diversity, but also make the linguistic results produced by the model appear increasingly outdated and irrelevant.
AI: Ethical and social consequences of textual incest
Finally, ethical and social considerations surrounding “textual incest” raise questions. The increasing homogenization of language and thinking through language models presents us with the challenge of reassessing the role of artificial intelligence in shaping public opinions and cultural norms.
This development could have a profound impact on our society, affecting not only the way we communicate, but also the way we think and understand our world. Against this background, the need for ethical reflection and regulation of AI technologies becomes clear to ensure that they are consistent with the values and norms of a society.
Hypothetical scenarios for clarity
Since these statements sound very abstract, I would like to illustrate them using a few hypothetical scenarios. In addition, a context should be created between the statements and possible questions. These scenarios are intended to demonstrate how language models, when in textual incest, could negatively impact development in various areas where language and texts play an important role.
First, let's look at message generation. Let's imagine a language model that uses its own AI-generated news articles for training. Such a model could get into a state where it starts producing repetitive content.
If you think about this scenario further, there is a possibility that this content will become increasingly disconnected from current events. This could ultimately lead to a distorted and potentially misleading picture of reality.
Impact in research and creative writing
Another hypothetical example concerns academic research. In a scenario where language models use AI-generated scientific papers for training, new research results could emerge based on untested and potentially incorrect interpretations by AI. This could create a vicious circle in which academic integrity and the quality of scientific knowledge suffers due to incorrect or distorted data.
Finally, I would like to address creative writing, which of course also plays an important role in my articles and columns. Here, excessive use of AI texts could lead to a homogenization of literary styles and themes.
Such a development would not only limit the diversity and breadth of literary expression, but could also contribute to stifling innovation and creativity in the field of creative writing.
This way, the risk of text incest in large AI language models can be minimized
So how can one deal with the risk of text incest in language models? Due to the complexity and multi-layered nature of language and the multitude of factors that influence language, in my opinion there is not just one strategy for avoiding text incest. Rather, it will be necessary to employ multiple proactive strategies.
A key approach to avoiding text incest is to diversify training data sources. The inclusion of human-written texts from different cultures, languages and subject areas leads to greater diversity of linguistic input. This diversity prevents the language model from becoming an echo chamber of its own results.
Another important approach is to regularly update the training data sets with current, real texts. This means that the language models remain adapted to the development of human language and social changes.
It is also necessary to include human supervision. Linguists, experts and ethicists in the training process help to identify and correct distortions, inaccuracies and ethical concerns in the model results.
Feedback mechanisms can also make a significant contribution to improving the models. They allow users to report errors or biases in AI-generated content, creating a feedback loop that contributes to continuous improvement of the language model.
Cross-model training
Cross-model training and benchmarking is also essential. Here, language models are trained not only based on their own results, but also on the results of other models, and their performance is compared with benchmarks created by humans in order to increase the diversity and reliability of AI texts.
The development and adherence to ethical guidelines and standards for language models also plays a particularly crucial role. This ensures the responsible use of AI, especially in sensitive areas such as news production and academic research.
Finally, maintaining transparency when dealing with AI is essential. Transparency regarding the sources and methods used in training language models promotes trust and enables external verification of quality and impartiality. This is crucial for the integrity and reliability of AI.
Conclusion: Text incest in AI language models
The phenomenon of so-called text incest in large language models such as ChatGPT, which is characterized by the use of AI texts as training material for language models, leads to a feedback loop that affects the diversity and accuracy of AI texts.
This poses risks such as the creation of echo chambers, loss of creativity and amplification of errors, which in turn can have a negative impact on the reliability of information and language development. There are also ethical concerns about the role of language models in society.
To minimize these risks, strategies such as diversification of training data sources, regular updates with current texts, human supervision, feedback mechanisms and ethical guidelines are required. In addition, the transparency of training methods is essential to ensure trust and quality assurance. These measures can be crucial to maintaining the integrity and diversity of AI texts.
Also interesting:
Source: https://www.basicthinking.de/blog/2024/01/26/textinzest-ki-texte-im-internet/