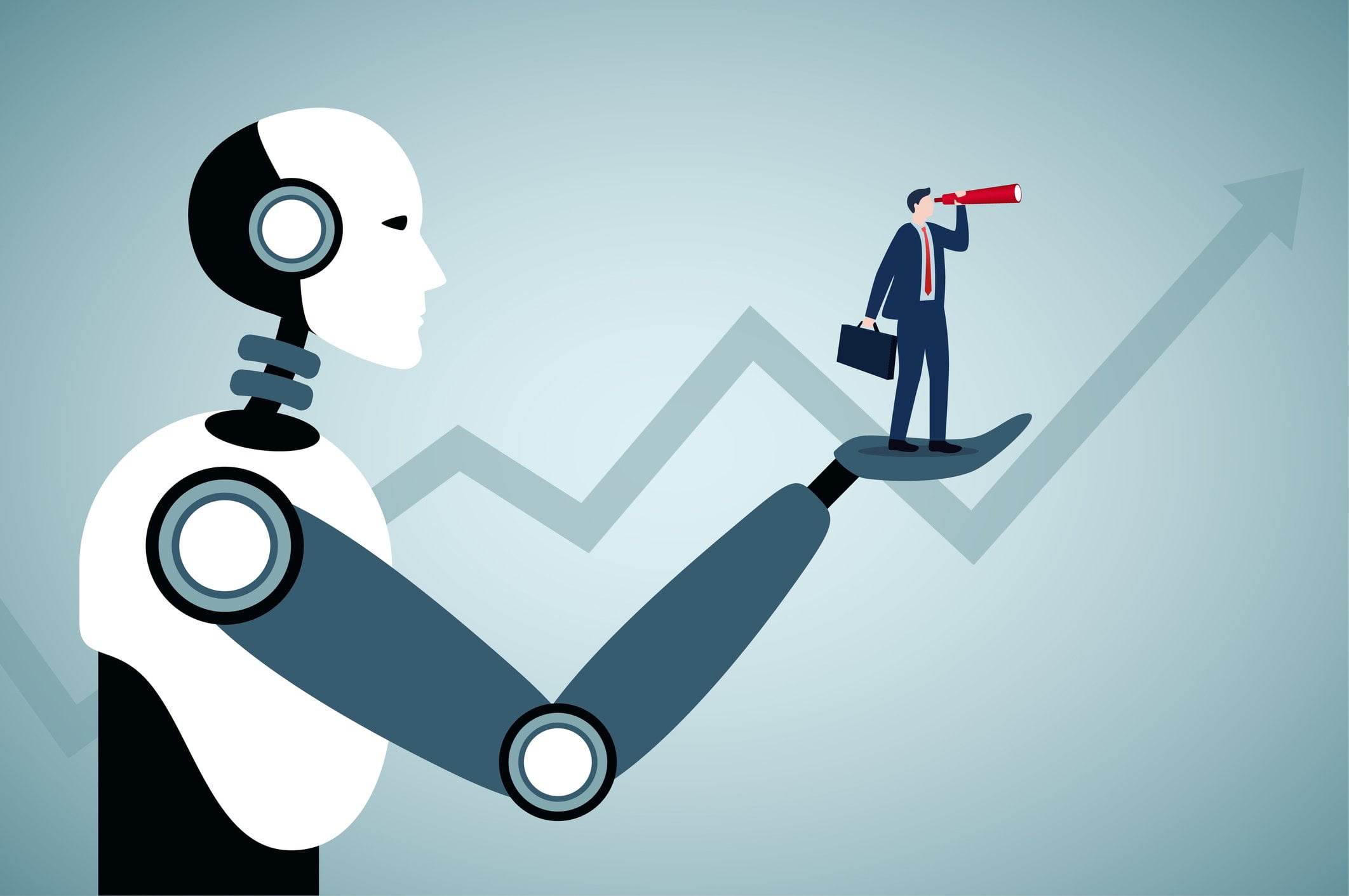
Alex Karp, CEO of the AI platform Palantir, has a clear message: “Now is the time to use artificial intelligence (AI) to write profits instead of poems.”
But how do leading companies in the field of artificial intelligence, many of them tech players from the USA, manage to seamlessly integrate AI into their existing processes and establish innovative business models?
Success factor 1: Involvement of top management and departmental leadership
While many companies often start the implementation of their first AI use cases from the ground up, widespread integration requires a mandate from the management. They must believe in the technology and provide the teams with the necessary support. Why? Well, the considerable investment requirements and the payback period until the initiatives have a noticeable impact on the company's success require patience, which makes the backing of top management indispensable.
In addition, the introduction and scaling of AI requires a cultural change towards a culture of experimentation in agile teams. Because it is precisely this mindset, this collaboration model, that leads to a higher risk tolerance and enables the exchange of knowledge – especially when the paths to the goal are not predefined. After all, AI is not just a technology for automating processes, but also offers companies the opportunity to establish completely new business models in the future – a key management task.
Read also
In addition to top management, the middle management Managers in the specialist departments have important knowledge regarding pain points and potentials as well as budgets and human resources. They are the ones who benefit when initiatives are piloted in their area and ultimately brought to maturity. This is precisely why data scientists should try to to win these managers as sponsorsThere is also an opportunity for middle management to distinguish themselves by engaging with AI and actively seeking contact with colleagues from IT and data science.
In startups, investors are often the drivers for the use of AI in order to increase the value of their investments with targeted investments. This momentum from the capital side should be used to advance relevant topics with concrete payback – not for token projects without a concrete business case.
Success factor 2: Collaborative organizational models
The aforementioned collaboration in agile teams of data scientists and business managers is the key to the successful implementation of AI use cases. These teams take permanent responsibility for business success and, because they combine different skills, they are fully capable of making decisions and taking action and bridge the classic challenges of functional organizations, such as complex coordination processes between departments or silo thinking.
Agile working methods enable teams to get used to each other and ultimately become experts for their customers and products. This is particularly crucial in the field of AI, as it is about developing the optimal models for solving problems through continuous experimentation. If the organization has little experience in using agile methods, a manager interested in technology with a tech sales or consulting background can initially mediate between the specialist departments and internal IT or even external service providers.
As artificial intelligence becomes more mature and relevant, insourcing and setting up different agile product teams for application areas such as CRM or forecasting is becoming more and more popular. Startups in particular often organize themselves into agile teams, as this is a key success factor for digital innovations of any kind.
Read also
It is more challenging for young companies that are at home in traditional industries and are not used to agile working methods. Or for those companies that have too few data science resources and therefore do not have the opportunity to distribute them across different product teams.
Success factor 3: Promote cultural change
Precisely because the successful development of AI models is like constant experimentation with uncertain outcomes, it is so important to have a To initiate a cultural change that promotes such a way of working. Let us no longer speak of a culture of error, but of a culture of experimentation. It is therefore precisely about minimising the costs of errors and creating a cultural and technological framework that is needed for such a view.
Today, more and more German companies – especially in the startup environment – are following the Anglo-Saxon model and defining principles that reflect values such as open communication, data orientation and sense of responsibility and performance To be credible and effective, these values must be exemplified from the top and reflected throughout the entire employee life cycle – from recruitment to feedback discussions and promotions. In this way, the willingness to take calculated risks should increase, but also the understanding should take hold that the automation of tasks does not release anyone from responsibility for the work result.
In addition to the long-term task of establishing a culture of innovation, the short-term to take employees’ fears seriouslyto make clear to them your important role in collaborating and training the AI co-pilots and to show them alternative roles and career paths. This communication should be accompanied by training opportunities for employees. Even though one might think that young companies have fewer challenges with such a cultural change than established large companies, or that they already have a corresponding mindset, this is by no means the case. I recently spoke to founders and managers of startups who are currently laboriously driving forward such a cultural change.
Success factor 4: Incorporating artificial intelligence into strategic decisions
As AI becomes increasingly important for companies, its influence on strategic decisions must also increase. An important factor here is the Conception of a data strategywhich includes a company's long-term vision for the collection, storage and use of its data. A frequently established concept here is a decentralized data architecture (data mesh), where data is managed and provided by specialized teams according to requirements according to clearly defined specialist areas (e.g. customers, suppliers). In this way, classic problems of big data architectures such as data silos or long response times are eliminated.
Read also
Another strategic consideration is the Rethinking business processes to make AI usable in the first place. In order to leverage the potential for business model innovation of artificial intelligence, it is necessary to question existing processes. One example of this is autonomous vehicles, which will lead to a change in vehicle architecture and production in the medium term and will massively strengthen business models such as peer-to-peer mobility platforms. However, in order not to slow down the AI transformation before it has gained momentum through strenuous process adjustments, the use cases that are most suitable for this should be chosen at the start. no Reengineering is necessary.
A particularly important strategic decision with regard to artificial intelligence is whether to develop solutions in-house or to outsource them (build or buy). Surprisingly, it is currently startups and digital natives who are increasingly integrating turnkey AI solutions in order to gain speed. At a later point in time, it will certainly make sense to consider in-house development, depending on factors such as the strategic relevance for the company, the differentiation potential of a use case or the availability of proprietary data.
Success factor 5: Create an environment for AI integration
A key factor for success is the implementation of routine procedures for measuring progress of AI transformation. First of all, a framework of key performance indicators is needed for data scientists that is aligned with the company's business goals. This is the only way experts can effectively pursue the overarching goals and the danger of AI becoming a research project with no connection to the business is averted.
These metrics usually focus on concrete business results such as increasing conversion. But measuring the progress of AI initiatives applies not only to the technology areas, but also to the processes in the business departments. AI pioneers increase their success by using metrics to measure AI adoption, such as automation rates, and closely tracking them in business reviews.
Success factor 6: Technological competence development
To start an AI transformation, system integrators can be commissioned to develop solutions or even integrate turnkey solutions. With increasing ambitions The importance of experienced data scientists is increasing for the successful development of AI models in the company. Because they bring valuable experience from completed projects and focus on delivering tangible business results. This also requires the establishment of central data engineering teams, as they ensure the continuous availability and quality of the required data.
Additionally, I would like to highlight the centralized “platform teams” as a key success factor. They provide reusable tools, blueprints and training to ensure that the application of artificial intelligence is possible beyond individual areas and across the entire company.
Another crucial aspect of developing your own models is the productization of AI use cases. This means that AI models can be used both internally in known applications (such as ERP or CRM systems) immediately usable and seamlessly integrated into commercially viable products for external customers. This requires particular attention because data scientists often lack the critical ability to productize, as this falls into the realm of traditional software development.
A final key driver for AI success in the scaling phase of mature AI users is the Democratization of AI. The goal here is to enable developers and business analysts without in-depth AI knowledge to independently develop AI solutions via low-code/no-code platforms. They enable companies to use AI even without extensive data science knowledge, so that AI spreads faster and scarce resources do not become a bottleneck.
This article was written by Dr. Tawia Odoi, member of the management board at Lidl Digital International, former manager at Amazon and AWS, author and speaker. He has recorded his experiences from research and business practice in his book “AI Excellence – Success Factors in Management Beyond the Hype”.
Read also
Source: https://www.businessinsider.de/gruenderszene/technologie/sechs-faktoren-um-ki-intelligenz-erfolgreich-in-eurem-unternehmen-zu-etablieren/