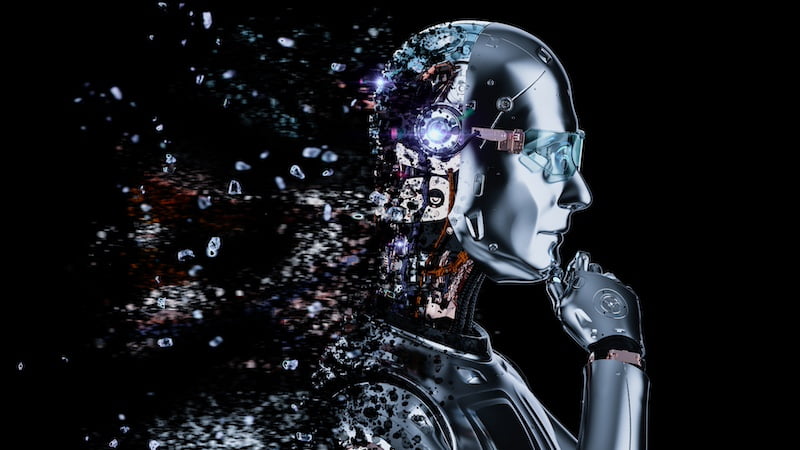
Artificial intelligence requires intensive training to develop further. But what happens when AI learns from AI? According to a new study, this has a negative impact because it causes AI systems to become increasingly worse.
An AI system needs huge amounts of data for its training. But what happens when this data is itself generated by AI? Researchers at Rice University in Houston, Texas, and Stanford University have been looking into this question.
In their study “Self-Consuming Generative Models Go MAD,” they concluded that AI systems perform worse when AI-generated images are used for their training.
What happens when AI learns from AI?
For their study, the researchers focused on image generators such as DALL·E 3 from OpenAI as well as Midjourney and Stable Diffusion.
Rapid advances in generative AI algorithms for images, text, and other data types have created a strong temptation to use synthetic data to train next-generation models.
According to the researchers, if AI-generated data is repeatedly incorporated into the training of new AI generations, an autophagic – “self-consuming” – loop is created. In their test, they exposed the various AI models to such autophagic loops.
As a result, both quality and variety suffered. Both characteristics decreased progressively. The researchers refer to this condition as Model Autophagy Disorder (MAD) in reference to mad cow disease.
Artificial intelligence generates hatched faces
For their study, the researchers trained AI models with different data. In each new stage, they relied more heavily on the images already generated by AI.
These already showed a complete synthetic loop in stage three, which resulted in cross-hatched artifacts in the images. In each subsequent generation, these artifacts increased. The researchers suspect that this may be an architectural fingerprint.
The less real data the AI models received, the more likely the images they produced were to be distorted. But cross-hatching was not the only thing that occurred.
This also resulted in data sets in which the people in the results became increasingly similar. In the end, some of them looked as if they were one and the same person.
AI could destroy itself
According to study author Richard Baraniuk, a professor at Rice University, this could have serious consequences for the development of artificial intelligence. AI systems could find themselves in a feedback loop after just a few generations through AI-based training.
This could cause the models to be “irreparably damaged”, which in turn would lead to the model collapse of the systems after only a short period of time.
Also interesting:
Source: https://www.basicthinking.de/blog/2024/08/08/studie-ki-lernt-von-ki/