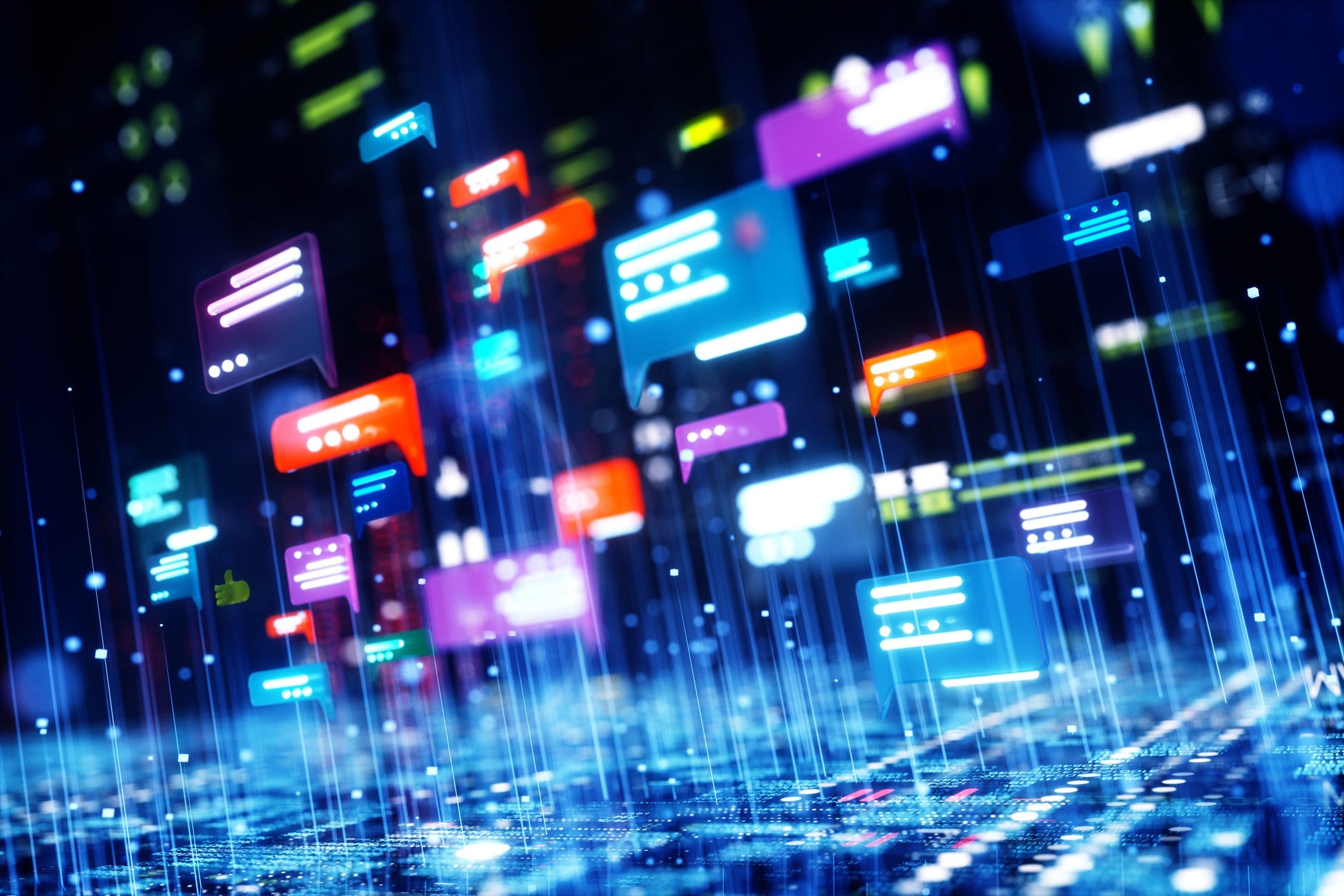
If you ask Google's artificial intelligence Gemini how many letters “R” are in the word strawberry, Gemini will happily announce, “The letter 'R' occurs once in the word strawberry.” When asked “Are you sure?”, Gemini answers According to: “If we go through the word 'strawberry' letter by letter, we only find a single 'R' in the second position. Strawberry. There are no other 'R's' in this word.” After that, Gemini would rather explain the best way to pick strawberries.
The whole thing would be funny if Gemini's inability to deal with numbers and letters didn't represent a fundamental problem with AI: large language models (LLM) often proclaim untruths with the tone of conviction. They particularly have problems with representing logical connections or numbers.
Read too
“Gemini is designed as a creativity and productivity tool and may not always be reliable,” said Google product boss Prabhakar Raghavan in the spring, commenting on the problems with Gemini. “There will be mistakes. As we've said from the start, hallucinations are a well-known challenge in all LLMs – there are cases where the AI just gets things wrong.” Especially with problems like these, it becomes abundantly clear how much the AI lacks some kind of worldview Every large language model only connects statistically probable words without questioning their meaning.
Invented wolf in logic puzzle
To the doctored riddle question, “I am standing on one side of the river with a raft and a goat. The raft can hold one person and one animal at a time, but if the goat is ever left alone on the opposite side, it will be eaten by a Komodo dragon. How can I get all my animals safely across the river in as few crossings as possible?”, even the latest AI from OpenAI, the language model “o1”, answers nonsense.
She invents a wolf, suggests several crossings, in short: o1 tries to adapt the reality of the question to its own limited training data: The AI knows the puzzle, but only with goat and wolf, the Komodo dragon confuses it.
Read too
The latest language model from OpenAI is actually optimized for logical thinking, is intended to justify its decisions and self-critically question whether they are coherent. It's not for nothing that the company's internal code name was “Strawberry” – the letter question for strawberries is dominated by o1. But o1 still hallucinates, especially when it comes to logic questions. Only when you criticize the answer does the AI realize that the wolf wasn't even part of the question.
But this demand could be built into the answer generation process: “One way to increase confidence in the results of LLMs is to support the results with arguments that are clear and easy to verify – a quality we call readability “says the research team led by neuroscientist Jan Henrik Kirchner, who conducted research at OpenAI to improve the reliability of the results.
AI always answers – even to impossible questions
They came up with the idea of training not one, but two artificial intelligences together. The first AI, the “prover,” generates an answer to a question. The second, the “examiner”, checks the result to see whether the solution is comprehensible.
Read too
“At the beginning of training there is no guarantee that the results will be error-free. But during training, the examiner becomes better at detecting errors,” the authors explain. This works particularly well with the logic problems, taken directly from secondary school mathematics lessons, which cause so many problems for AI.
However, a fundamental problem with AI is that the training of algorithms always aims to generate an answer, regardless of whether the AI knows the necessary training material or not. Anyone who runs Google's English-language AI-assisted search for the space program of the Austro-Hungarian Empire will receive the following AI summary of the search results: “In 1889, Austria-Hungary carried out its first manned orbital flight with a liquid-fuel rocket launched from the Galicia region.”
Read too
In addition, Google's AI happily continues to fantasize, the Habsburgs sent an expedition with 30 astronauts to Mars in 1908, where they “set up a temporary research outpost and stayed for a year.”
This is funny at first glance. In the second, it becomes clear that the AI is mixing real history with science fiction in the compulsive attempt to answer the impossible question. The error is obvious.
Law firm experiment: Lawyers have to iron out AI errors
But what if you give the AI tasks that are normally done by specialist lawyers or engineers? Then it takes specialist lawyers or engineers to discover potentially very expensive errors: When a large German law firm tried using OpenAI's AI assistants based on GPT4o, the conclusion was sobering: the AI failed to draw up even simple legal letters correctly and made mistakes in the wording similar definitions, disappointed in setting up standard writing.
“I would have beaten the hell out of any trainee,” says one of the lawyers involved, who wishes to remain anonymous. But only a specialist lawyer can recognize these errors as such: “This shows a classic problem with the use of AI: If you cannot judge something, you draw conclusions from the outside to the inside and may be wrong. Anyone who lets ChatGPT write contracts without any knowledge will receive a plausible and good-looking document. He just might not realize that it’s objectively not good.”
Read too
The German AI pioneer Aleph Alpha shows an approach to solving the hallucination problem with its new AI operating system Pharia. “Hallucinations occur because, despite all the developments, AI systems still do not truly understand our world,” explains Aleph Alpha CEO Jonas Andrulis. “This is particularly true for topics that the models do not know from their training, for example in specific specialist areas such as logistics or compliance. The common systems on the market are simply a bit more condensed.”
Aleph Alpha relies on syllables instead of words – and lets AI ask for help
Aleph Alpha's new AI system has two possible solutions: Firstly, the researchers have found a way to teach the models technical terms afterwards. “We have developed a method that does not require us to use a defined, restricted vocabulary at all,” explains Andrulis.
To put it simply, Aleph Alpha trains its model not with words, but with word syllables. This makes it relatively easy to introduce new terms into German. “Our new system also supports a function that we call ‘catch’,” says Andrulis.
Read too
This function is intended to capture the knowledge of a company's experts, transform it into the AI database and make it usable company-wide. “If the algorithm determines that it does not have sufficient knowledge about a question, it can actively ask experts in the company for help.” The AI remembers the experts’ answers and uses them for all subsequent questions. However, this training is limited to individual companies and specific topics.
It doesn't solve the fundamental hallucination problem. The specialist knowledge remains within the company and is not incorporated into the general knowledge of a large model. That should be just fine with Aleph Alpha customers.
This article first appeared on Welt.de.
Source: https://www.businessinsider.de/gruenderszene/technologie/kuenstliche-intelligenz-im-irrtum-waehrend-google-und-openai-noch-suchen-will-aleph-alpha-die-loesung-gefunden-haben/